Adaptive Learning to Personalized Learning
Nowhere is artificial intelligence more prominent or controversial in education than in personalized learning.
Computer driven adaptive learning has been around for decades; in its most basic form it is simply the computer program branching the learning path based upon responses the student makes. Some learners may be best served by materials delivered in a different format, for example case studies rather than theoretical study. Others may need refresher learning for underpinning skills, principles, and theories upon which more advanced learning is built. Computer Assisted Instruction (CAI) has enabled this kind of learning program for half a century and more. I recall working with others in the 1970’s as we programed simple lessons that would quiz students and branch their learning path based on right answers as well as wrong answers. Simple coding in the PLATO TUTOR language would allow programmers to branch to different review or new materials based upon which answer was selected.
More sophisticated adaptive learning programs that have been developed recently aggregate much more data from the learner to better adapt the learning path. These data can include stored prior learning experiences and performances; student self-expressed preferences in modes of delivery; analytical prediction of likelihood of success for the individual student through different modes of delivery; and much more. For the past half dozen years, Khan Academy has developed and enhanced their flow of learning model. These and other like programs can more finely and accurately identify and address gaps in learning. Coupled with effective support modules, they can fill in the gaps on an individualized basis. “Particularly in high-enrollment classes, adaptive learning can provide tailored support and guidance to all students,” says this primer from EDUCAUSE. Adaptive learning has effectively been used by many publishers for their online homework and supplementary materials.
Adaptive learning, while it has provided an important step forward in helping to assure that all learners get the material that they need to achieve learning outcomes, has fallen short in cultivating full engagement with the individual student. That’s where personalized learning takes the next step. It is defined by the Glossary for Education Reform as:
The term personalized learning, or personalization, refers to a diverse variety of educational programs, learning experiences, instructional approaches, and academic-support strategies that are intended to address the distinct learning needs, interests, aspirations, or cultural backgrounds of individual students. Personalized learning is generally seen as an alternative to so-called “one-size-fits-all” approaches to schooling in which teachers may, for example, provide all students in a given course with the same type of instruction, the same assignments, and the same assessments with little variation or modification from student to student.
This takes student-centered learning to the next level. It goes beyond simply responding to requests from students. Instead, students become part of the process of defining the learning outcomes, pedagogy, and practices of the learning experience. Until recently, it has not seemed feasible to meet student needs in this way. To customize learning for each of 30 or 40 students in a class, monitor their individual progress, and provide meaningful feedback just is too time consuming.
Now, machine learning can synthesize the huge volume of data needed to more fully deliver student-centered learning. It can assemble the background; take input from the individual learner regarding their self-determined needs and expectations; identify learning deficits and needs; and produce and present the learning path to best accomplish those goals.
In this case, the role of the faculty member shifts from directly delivering materials and grading based on a single syllabus to advising, assisting, and assessing personalized learning that meets the needs of both the individual and the prescribed outcomes of the program. Certainly, this is a change for the faculty member. It is no longer administering a “one-size-fits-all” class. Instead it is a much more personal, individualized mentoring of each of the students while AI assembles the learning stack for each student.
Have you incorporated any of the adaptive learning tools in your classes? Are you preparing for the next step of personalized learning? Are you preparing your faculty colleagues for this process?
This article originally appeared in Inside Higher Ed’s Inside Digital Learning Blog.
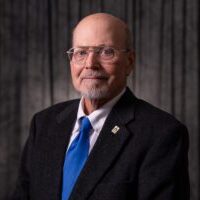
Ray Schroeder is Professor Emeritus, Associate Vice Chancellor for Online Learning at the University of Illinois Springfield (UIS) and Senior Fellow at UPCEA. Each year, Ray publishes and presents nationally on emerging topics in online and technology-enhanced learning. Ray’s social media publications daily reach more than 12,000 professionals. He is the inaugural recipient of the A. Frank Mayadas Online Leadership Award, recipient of the University of Illinois Distinguished Service Award, the United States Distance Learning Association Hall of Fame Award, and the American Journal of Distance Education/University of Wisconsin Wedemeyer Excellence in Distance Education Award 2016.
Other UPCEA Updates + Blogs
Whether you need benchmarking studies, or market research for a new program, UPCEA Consulting is the right choice.
We know you. We know the challenges you face and we have the solutions you need. We speak your language and have been serving leaders like you for more than 100 years. UPCEA consultants are current or former continuing and online higher education professionals who are experts in the industry—put our expertise to work for you.
UPCEA is dedicated to advancing quality online learning at the institutional level. UPCEA is uniquely focused on excellence at the highest levels – leadership, administration, strategy – applying a macro lens to the online teaching and learning enterprise. Its engaged members include the stewards of online learning at most of the leading universities in the nation.
We offers a variety of custom research options through a variable pricing model.